(Statistical) Analysis plan
A (statistical) analysis plan, ‘SAP’ for short, describes how the quantitative or qualitative data that you will collect will be statistically handled. You may add it as a supplement to your protocol.
On this page:
What is a SAP?
A SAP a is a more technical document than the research protocol and includes detailed procedures for executing statistical analyses. Although a SAP was originally intended for clinical trials, also other types of research design may profit from transparent analysis plans. For example, the analysis of qualitative data is likely to benefit from a written plan, describing the underlying (philosophical) approach and tackling details of e.g. triangulation, criteria for saturation and the selection of quotes.
Why write a SAP?
- Developing a SAP forces you to think about which data to collect in which format, which may then guide your decisions on e.g. measurement instruments and timing of (repeated) measurements. It may also alert you to the fact that you may be planning to collect more data than you will be using in your analyses. This may burden participants and cause them to (selectively) drop out, which may jeopardize the overall validity of your study. Here is a link to a framework that helps you select the minimally needed set of confounding factors for a valid data-analysis. A recent example taught us that the statistical repair of bias due to poor treatment adherence in a trial would have required repeatedly measuring time-dependent confounders. Unfortunately, this had been forgotten and adjustment for adherence became impossible. Note that collecting personal data unnecessarily is unlawful.
- SAP development may alert you that the necessary tools or statistical techniques are not available (in your favorite software). SAP development may also flag up that (more) statistical support needs to be organized.
- A good SAP, and sticking to it, may save you a lot of time that would otherwise be spent analyzing the data in haphazard and data-driven ways (it prevents “data-dredging” where data are selected based on desired outcomes).
- A good reason to put your SAP along with your research protocol in the public domain early on is that the desire (unfortunately far from eradicated) to produce statistically significant results leads many investigators to torture their data until they confess (P-hacking ). This approach distorts your work (then reviews, guidelines and patient care or services, and the global scientific record as a whole) and should be avoided at all cost. This issue is explained in more detail in the chapter on preregistration .
- Given the influence of statistical decisions on study conclusions, well-documented and transparent statistical conduct is essential.
How to write a SAP?
Crystal clear research objectives guide every data analysis. Before embarking on thinking about the analysis, revisit your research objectives and consider asking some of your colleagues, not directly involved in the project, to give their feedback. After all, some tunnel vision is common in many project teams. Each paper you write may require a separate SAP or an adaptation of the main SAP. To describe the exact contents of SAPs for all the different research designs is beyond the scope of this chapter, which only provides you rough guidance, not a format for each conceivable design. But please consult the reporting guidelines at equator network . They list many study design and analysis items you can use in a SAP or study protocol. More guidance on SAPs for non-trials may be found in PubMed by e.g. searching with this string: “statistical analysis plan” NOT (randomized OR randomised OR trial) or slight variations of it. Increasingly, you may find example SAPs in Open Science Framework (search with e.g. “statistical analysis plan”). Below we provide some more guidance, tips and tricks.
Phases in statistical analysis
We follow Rothman, Greenland and Lash (Modern epidemiology, 3rd edition) and distinguish the following phases in a statistical analysis that should all be thought out and described beforehand:
- Data editing (check for accuracy (detecting impossible values, looking at distributions), consistency (impossible combinations in related variables)) and completeness (missing values)), matrix scatterplots for continuous variables (detecting bivariate outliers)
- Data description and summarization (intelligently chosen tables, histograms, scatterplots, etc.) choosing (logical) categories (without regard to test statistics to avoid bias and avoiding thoughtless use of tertiles, quartiles or quintiles, but instead focusing on (biological) meaning and optimal contrasts)
- Handling of missing values (the possible use of multiple imputation)
- Estimation (simple tabulation, (simple) stratified analyses, (multiple) regression)
- Sensitivity and influence analyses (robustness) describing how you will explore the effect of potential violations of assumptions and/or results depending on a few particular observations (subjects)
- More advanced quantitative bias analysis.
Recommended items in a (clinical trial) SAP
Whatever study you are planning or conducting, look here for excellent guidance on what should be in a SAP for a clinical trial. But even if you use another research design, many of the principles for trials still apply or can be a great source of inspiration. After all, missing data often occur and sensitivity analyses should always be considered given the multitude of subjective decisions involved in most (statistical) analyses. A good way to structure section 6 of the above document is this:
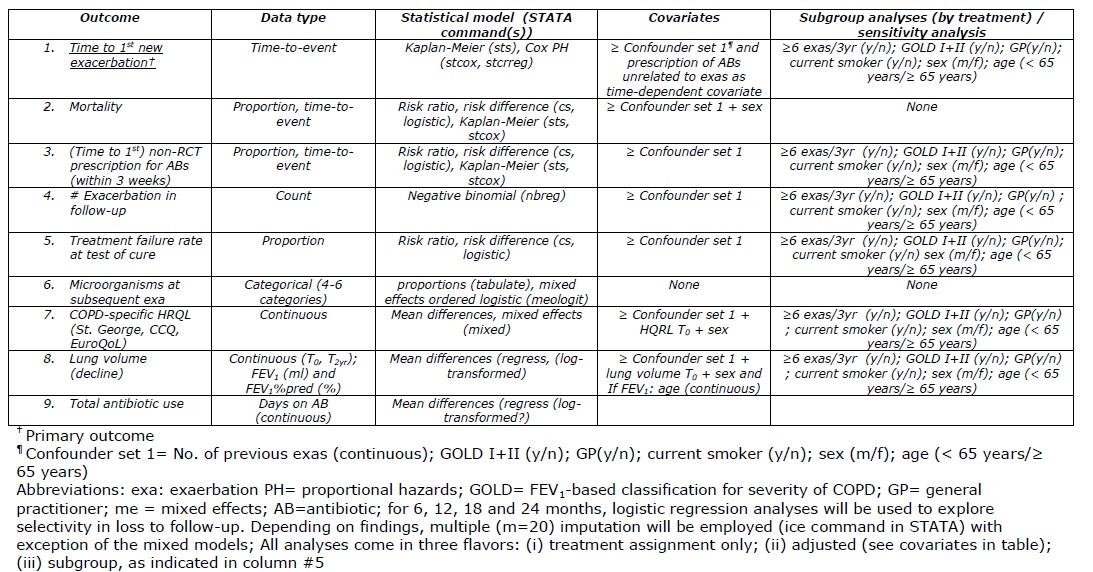
Or this:
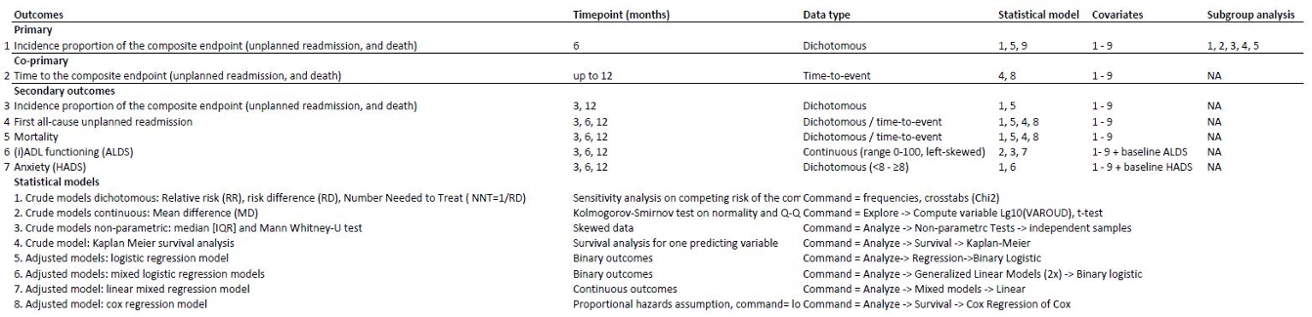
Dealing with adverse effects in the analysis
The collection and analysis of harms or adverse effects (in trials) is a particularly underdeveloped topic. Guidance on how to deal with adverse effects in the analysis may be found here .
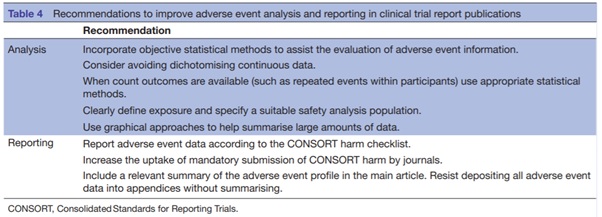
Other principles
- Think deeply about and then design skeleton tables. Skeleton tables have a title, row labels and the column entries are exactly spelled out, only the numbers in the cells are lacking. The data analysis will produce the contents of the cells in a very targeted way. That is, hardly any other numbers need to be produced. The same principle applies to graphs.
- Thoroughly discuss your SAP early on with your team members and make sure there is team consensus about the main issues and document decisions made (with reasons) in your logbook or meeting minutes. It can be quite frustrating to conduct many analyses only to be sent back to your computer by the project leader to redo the work because s/he wants to shift the emphasis of the analyses.
- Try to prepare most of the syntax (code) before the data collection is complete or before the analyses start. This will also motivate you to think about (short) variable names. Why call a variable ‘randomization_group’ if it may be called ‘trt’ (for treatment) or ‘intv’ (for intervention). A variable name is not a variable label!
What to do if I preregistered my SAP and I want to change it?
See chapter on preregistration .
More information
If you need tailored advice (beyond randomized trials), please write an email to opensciencesupport@hva.nl. They can help you with developing a SAP for a quantitative as well as for a qualitative or mixed methods study. Further reading: